Hi, Can you believe that Dutch painter Vincent Van Gogh only sold one artwork during his lifetime? It wasn't until years after his death that he became the admired Post-Impressionist genius known for his striking colors and emphatic brush strokes. Yet today, art students study Van Gogh, and artists create replicas of his masterpieces. If only everyone had that kind of talent... What if you could create a masterpiece like Van Gogh's — even if you're not an artist? In fact, you could even transform any photo into a make-believe Van Gogh painting for social media. Mimicking art masterpieces is just one of the tasks Generative Adversarial Networks, or GANs for short, are capable of. The possibilities of what we can create with GANs are as boundless as Van Gogh's imagination. In this post, you will get an overview of how GANs work at a high level, a few GAN variants with breakthroughs, and the GAN applications for solving real-world problems. 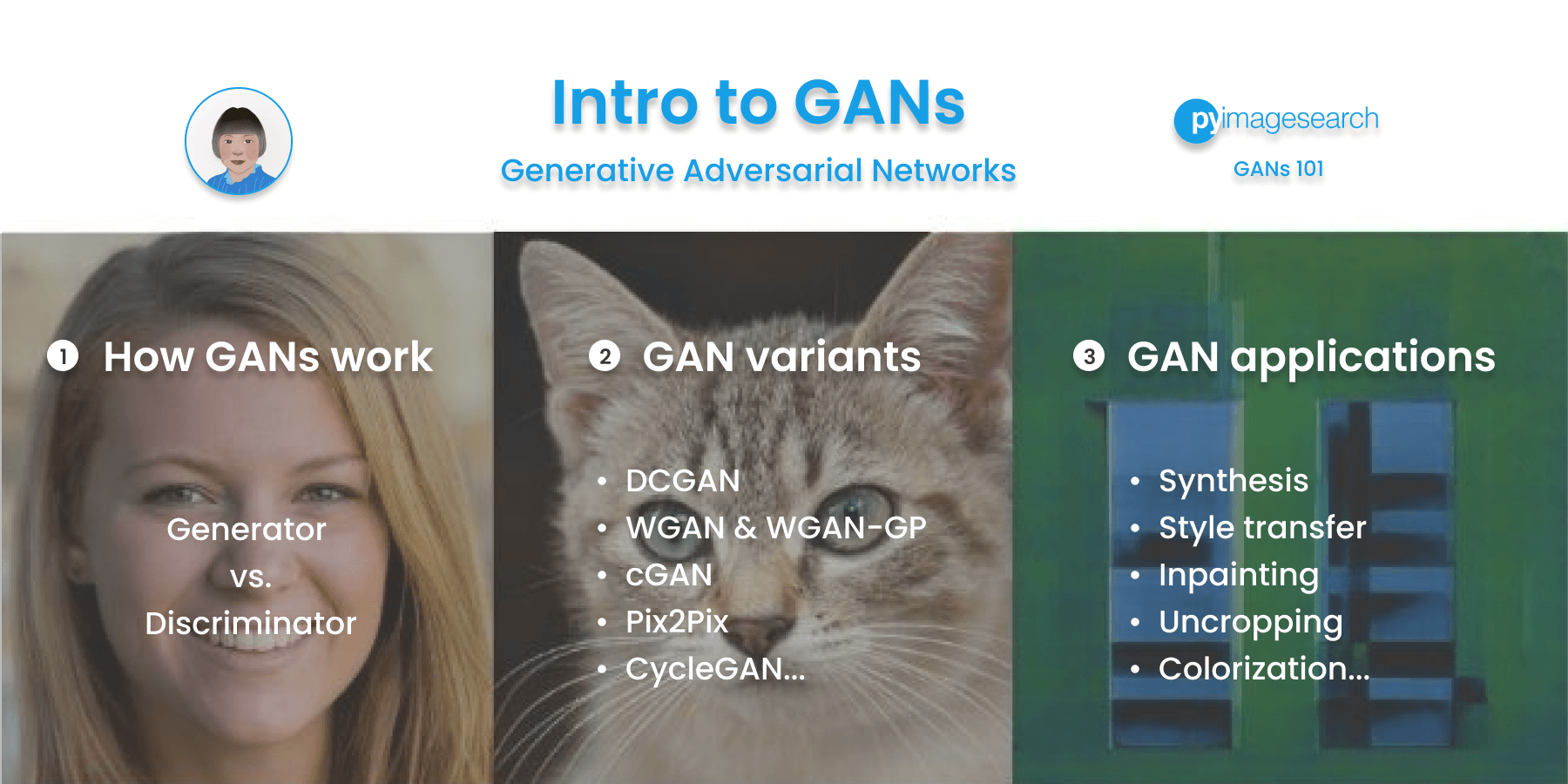 The big picture: GANs are able to generate new images by training two competing algorithms (a generator and a discriminator) simultaneously. How GANs work: - The generator creates images that look like the training input data
- The discriminator spots whether it is real (the training data) or fake (the generator's output)
- The generator and the discriminator both improve during training with feedback from each other
- Repeat until both the friendly competition between the generator and discriminator reaches an equilibrium: neither player can improve further.
Brilliant, isn't it? My thoughts: GANs can be used in various applications, from image/music/video synthesis, style transfer, to colorization — your imagination is the limit. You can even describe what you want to see, and the algorithm will generate a high-quality image for you (text-to-image generation/synthesis). We've seen many GAN advances since its inception in 2014, and we will continue to see GANs moving from research labs to solving real-world problems. Yes, but: GANs can generate completely new images that never existed before. But to be able to train a stable GAN is still very challenging. Stay smart: A good GAN model should have good image quality and diversity: a good variety of images get generated that approximate the distribution of the training dataset. GANs and their variants are fun, powerful, and versatile. While this post explores GAN applications mainly in art and design, GAN can also be applied to many other fields such as video games, medicine, agriculture, and climate change. The possibilities are endless. Click here to learn more about GANs PyImageSearch University This lesson is part of a new GANs course of PyImageSearch University, our flagship program to help you master computer vision, deep learning, and OpenCV. PyImageSearch University is updated each week with new courses. The GANs course is created by Margaret Maynard-Reid, an ML research engineer and artist, with previous experience on the computer vision team of Microsoft AI & Research and the University of Washington. She is also recognized for her ML expertise as a Google Developer Expert (GDE). Don't know Python? No problem, we've got you covered with a short and sweet Python course to get you going. Having problems with your local development environment or IDE? Great, because our pre-configured Colab Notebooks mean you can run code the moment you join PyImageSearch University. You don't want to be a sys-admin, so don't waste your time messing with your development environment. You can find the current lesson under GANs 101. The direct link to the lesson can be found here. Want to master computer vision and deep learning? Do you think mastering computer vision and deep learning has to be time-consuming, overwhelming, and complicated? Or has to involve complex mathematics and equations? Or requires a degree in computer science? That's not the case. All you need to master computer vision and deep learning is for someone to explain things to you in simple, intuitive terms. And that's exactly what I do. My mission is to change education and how complex Artificial Intelligence topics are taught. Inside PyImageSearch University, you'll find: - 25 courses on the hottest computer vision, deep learning, and OpenCV topics
- 25 Certificates of Completion (one for each course)
- 37+ hours of on-demand video
- Pre-configured Jupyter Notebooks running in Google Colab
- Run all code examples in your web browser — works on Windows, macOS, and Linux (no dev environment configuration required!)
- Access to centralized code repos for all 400+ tutorials on the PyImageSearch blog
- Easy one-click downloads for code, datasets, pre-trained models, etc.
- Access on mobile, laptop, desktop, etc.
- Brand new courses released every month, ensuring you can keep up with state-of-the-art techniques
Click here to join PyImageSearch University Adrian Rosebrock Chief PyImageSearcher P.S. If you're interested in learning how to successfully apply deep learning to your own projects, I would recommend reading my book, Deep Learning for Computer Vision with Python. I crafted my book so that it perfectly balances theory with implementation, ensuring you properly master: - Deep learning fundamentals and theory without unnecessary mathematical fluff. I present the basic equations and back them up with code walkthroughs that you can implement and easily understand. You don't need a degree in advanced mathematics to understand this book
- How to implement your own custom neural network architectures. Not only will you learn how to implement state-of-the-art architectures, including ResNet, SqueezeNet, etc., but you'll also learn how to create your own custom CNNs
- How to train CNNs on your own datasets. Most deep learning tutorials don't teach you how to work with your own custom datasets. Mine do. You'll be training CNNs on your own datasets in no time
- Object detection (Faster R-CNNs, Single Shot Detectors, and RetinaNet) and instance segmentation (Mask R-CNN). Use these chapters to create your own custom object detectors and segmentation networks
You'll also find answers and proven code recipes to: - Create and prepare your own custom image datasets for image classification, object detection, and segmentation
- Hands-on tutorials (with lots of code) that not only show you the algorithms behind deep learning for computer vision but their implementations as well
- Put my tips, suggestions, and best practices into action, ensuring you maximize the accuracy of your models
If you're interested in learning more about my deep learning book, I'd be happy to send you a free PDF containing the Table of Contents and a few sample chapters: Click here to download your table of contents and sample chapters PDF After clicking the link above, the PDF will land in your inbox in a few short minutes. |
No comments:
Post a Comment
Note: Only a member of this blog may post a comment.