Hi, By this point, we all are familiar with Christopher Nolan's 2010 masterpiece "Inception." It was a fantastic movie that explored the themes of the subconscious and reality. The characters in the film procured a dream invasion machine that was created for military training through dreams and repurposed it for their own devious plans, which was to obtain information from people through their dreams. 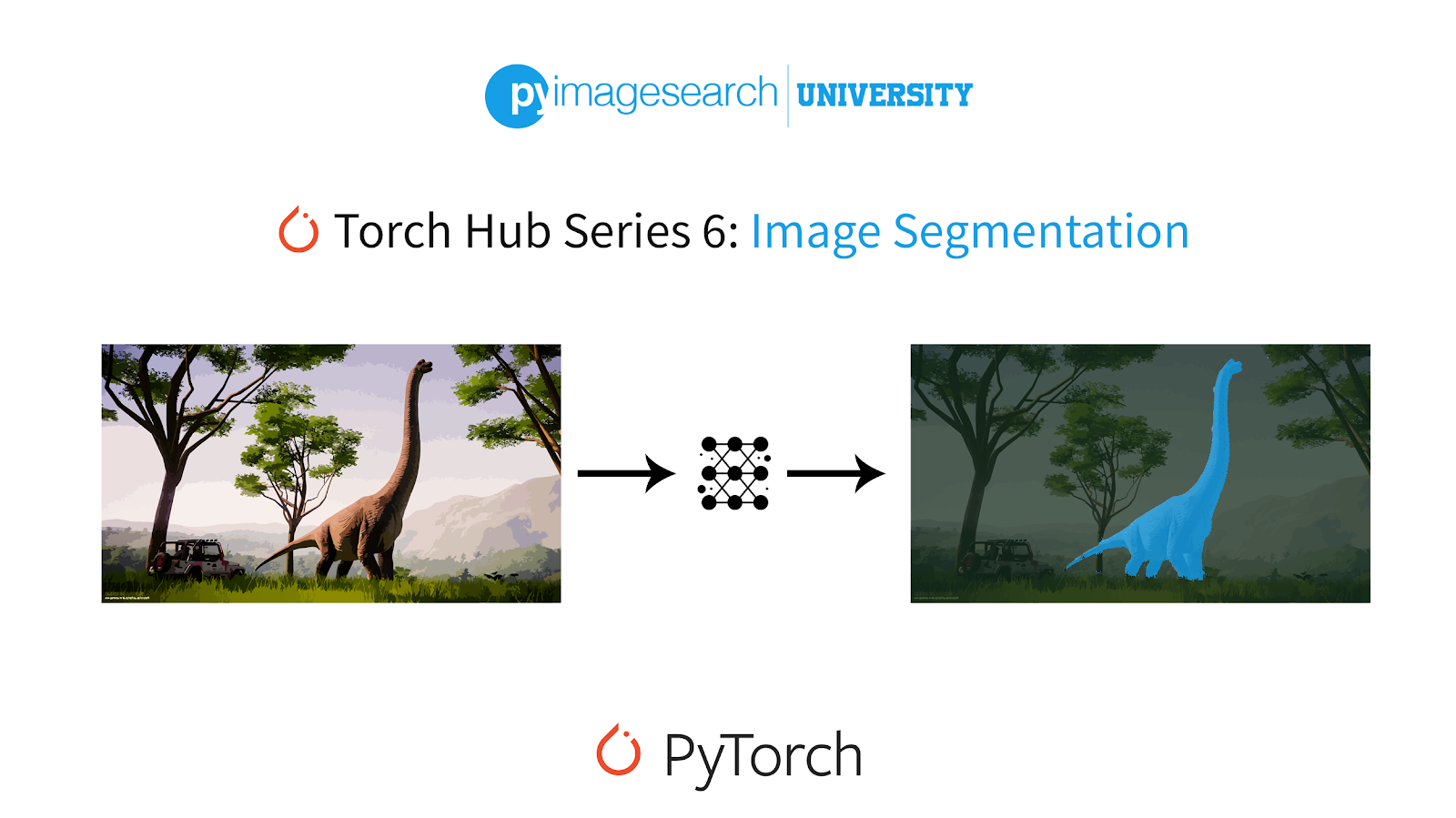 This begs the question of how it is related to today's post by Shivam Chandhok? You see, our spotlight today is the FCN (Fully Convolutional Network) by PyTorch. The idea behind this model is fairly simple; pre-trained classification models are repurposed to create image segmentation models. To reiterate, we are grabbing the weights of a model designed to handle a particular task and repurposing them to tackle a new problem. Since the pre-trained classification models are already adept feature extractors, changing their end goal labels to image segmentation yields fairly strong results. In addition, segmentation retains the need to extract the features of an image, much like the dream invasion machine of Inception, where the core need of access to dreams is needed, no matter what task you wish to do. With the power of pre-trained feature extractors trained on benchmark datasets, the FCN has produced some truly wonderful results. The big picture: Pre-trained classification models have been repurposed to create segmentation models. How it works: We remove the final FC layers and create a fully convolutional network for segmentation using deconvolutional layers for decoding. FCN reuses features learned during classification. Our thoughts: We use pre-trained FCN models from Torch Hub and seamlessly integrate them in our pipelines for real-time segmentation. Yes, but: Torch Hub supports limited models and does not have an FCN model for other fancier classification encoders. Also, models are pre-trained on limited datasets. Stay smart: Don't rely too much on Torch Hub. Instead, learn the salient architectural details and the prerequisites to fine-tune models on novel classes that the pre-trained FCN models have not been trained on already. Click here to read the full tutorial PyImageSearch University This lesson is part of PyImageSearch University, our flagship program to help you master computer vision, deep learning, and OpenCV. PyImageSearch University is updated each week with new lessons. Don't know Python? No problem, we've got you covered with a short and sweet Python course to get you going. Having problems with your local development environment or IDE? Fortunately, our pre-configured Colab Notebooks allow you to run code the moment you join PyImageSearch University. But, of course, you don't want to be a sys-admin, so don't waste time messing with your development environment. You can find the current lesson under Torch Hub 101 — Practical Applications of Torch Hub and the direct link here. Want to master computer vision and deep learning? Do you think mastering computer vision and deep learning has to be time-consuming, overwhelming, and complicated? Or has to involve complex mathematics and equations? Or requires a degree in computer science? That's not the case. All you need to master computer vision and deep learning is for someone to explain things to you in simple, intuitive terms. And that's exactly what we do. Our mission is to change education and how complex Artificial Intelligence topics are taught. Inside PyImageSearch University, you'll find: - 30 courses on the hottest computer vision, deep learning, and OpenCV topics
- 30 Certificates of Completion (one for each course)
- 39+ hours of on-demand video
- Pre-configured Jupyter Notebooks running in Google Colab
- Run all code examples in your web browser — works on Windows, macOS, and Linux (no dev environment configuration required!)
- Access to centralized code repos for all 500+ tutorials on the PyImageSearch blog
- Easy one-click downloads for code, datasets, pre-trained models, etc.
- Access on mobile, laptop, desktop, etc.
- New courses released regularly and new lessons weekly, ensuring you can keep up with state-of-the-art techniques
Click here to join PyImageSearch University PyImageSearch Team P.S. If you're interested in learning how to successfully apply deep learning to your own projects, I would recommend reading my book, Deep Learning for Computer Vision with Python. I crafted my book so that it perfectly balances theory with implementation, ensuring you properly master: - Deep learning fundamentals and theory without unnecessary mathematical fluff. I present the basic equations and back them up with code walkthroughs that you can implement and easily understand. You don't need a degree in advanced mathematics to understand this book
- How to implement your own custom neural network architectures. Not only will you learn how to implement state-of-the-art architectures, including ResNet, SqueezeNet, etc., but you'll also learn how to create your own custom CNNs
- How to train CNNs on your own datasets. Most deep learning tutorials don't teach you how to work with your own custom datasets. Mine do. You'll be training CNNs on your own datasets in no time
- Object detection (Faster R-CNNs, Single Shot Detectors, and RetinaNet) and instance segmentation (Mask R-CNN). Use these chapters to create your own custom object detectors and segmentation networks
You'll also find answers and proven code recipes to: - Create and prepare your own custom image datasets for image classification, object detection, and segmentation
- Hands-on tutorials (with lots of code) that not only show you the algorithms behind deep learning for computer vision but their implementations as well
- Put my tips, suggestions, and best practices into action, ensuring you maximize the accuracy of your models
If you're interested in learning more about my deep learning book, I'd be happy to send you a free PDF containing the Table of Contents and a few sample chapters: Click here to download your table of contents and sample chapters PDF After clicking the link above, the PDF will land in your inbox in a few short minutes. |
No comments:
Post a Comment
Note: Only a member of this blog may post a comment.